The rise of AI-powered text-to-video technology is revolutionizing the way we create and consume content. At the forefront of this transformation are the powerful Hugging Face models, which are rapidly becoming the go-to tools for content creators and businesses alike.
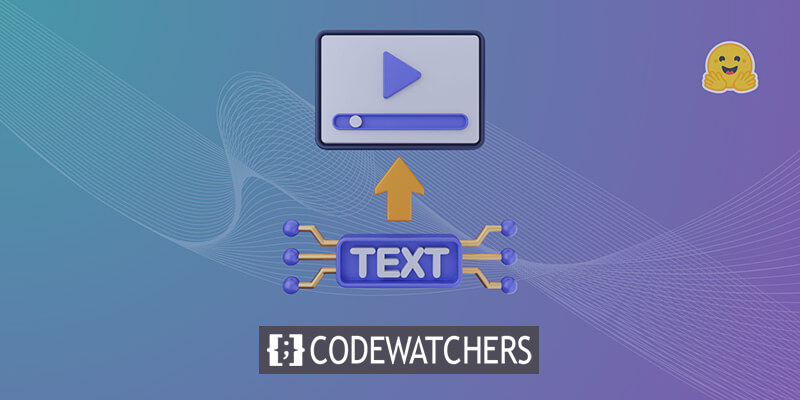
These state-of-the-art language models, trained on vast troves of data, possess the remarkable ability to translate written text into captivating visual narratives. By leveraging the latest advancements in natural language processing and generative AI, Hugging Face models can effortlessly transform your words into engaging, high-quality videos that captivate your audience.
Understanding Text-to-Video Technology
Text-to-video models transform written descriptions into moving images. These models understand text and convert it into a sequence of frames that depict the scene or action described. This process involves multiple steps, including text analysis, visual content generation, and frame sequencing. Each step requires complex algorithms to ensure the output video accurately represents the input text.
The journey of text-to-video technology began with simple text descriptions generating basic animations. Early models focused on creating static images, but advancements in AI and machine learning have enabled the development of dynamic video generation. Over the years, researchers have integrated sophisticated neural networks, leading to significant improvements in video quality and realism. These advancements have opened new possibilities for creative content creation and various applications across industries.
5 Best Text To Video AI Models From Huggingface
Here we have the 5 best AI text-to-video models from huggingface. These models are very popular for their distinct functionality and have the most number of downloads.
ModelScope - 1.7b
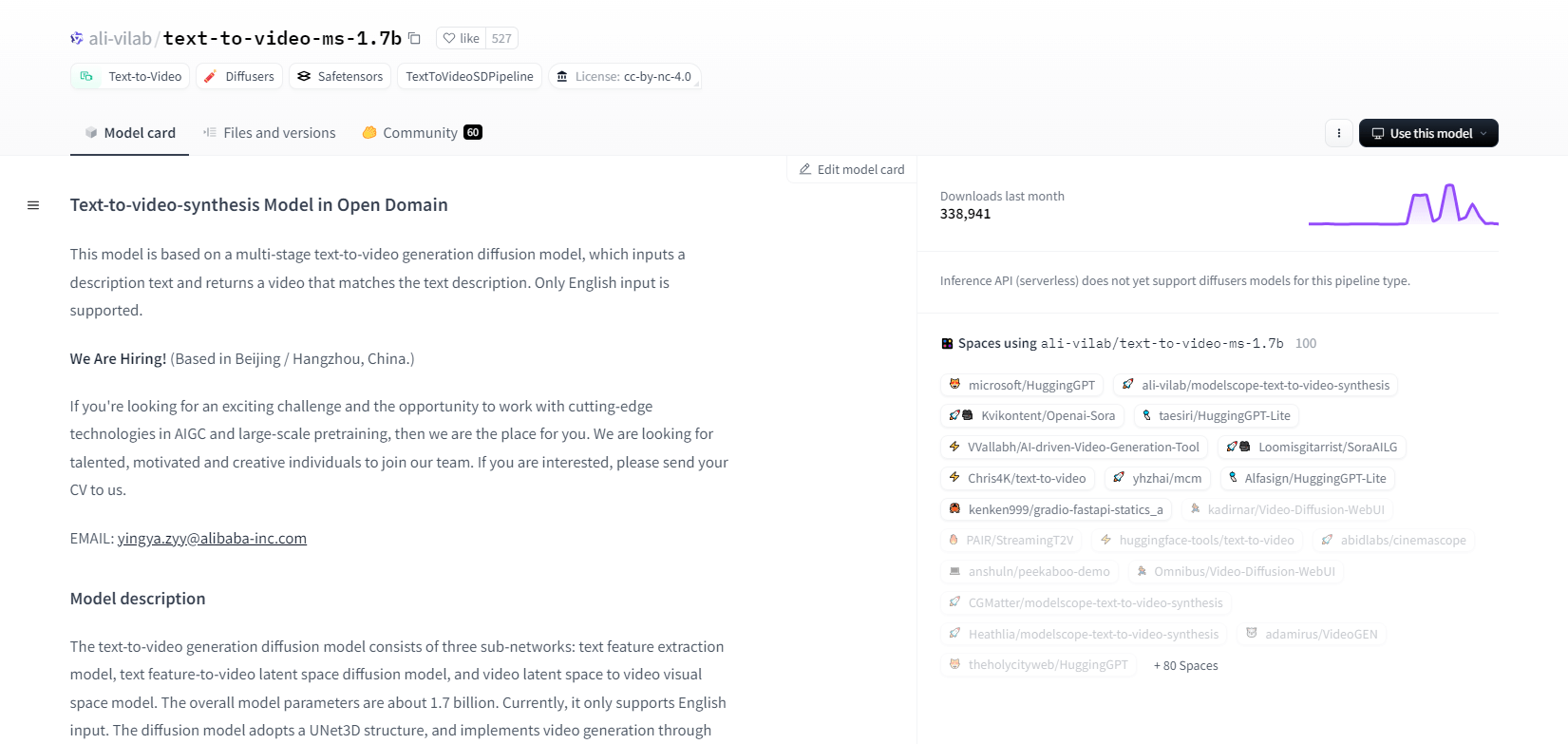
The text-to-video synthesis model from ModelScope uses a multi-stage diffusion process to generate videos from text descriptions. This advanced model supports only English input and is designed for research purposes. It consists of three sub-networks: text feature extraction, text-to-video latent space diffusion, and video latent space to visual space mapping.
With 1.7 billion parameters and a UNet3D structure, it iteratively denoises Gaussian noise to create videos. This model is suitable for various applications, like creating videos from arbitrary English text. However, it has limitations, such as biases from training data and an inability to produce high-quality film-level videos or clear text. Users must avoid generating harmful or false content. The model was trained on public datasets, including LAION5B, ImageNet, and Webvid.
Key Features
- Multi-stage diffusion process
- English text support
- 1.7 billion parameters
- UNet3D architecture
- Iterative denoising method
- Research purpose focus
- Public dataset training
- Arbitrary text generation
AnimateDiff-Lightning
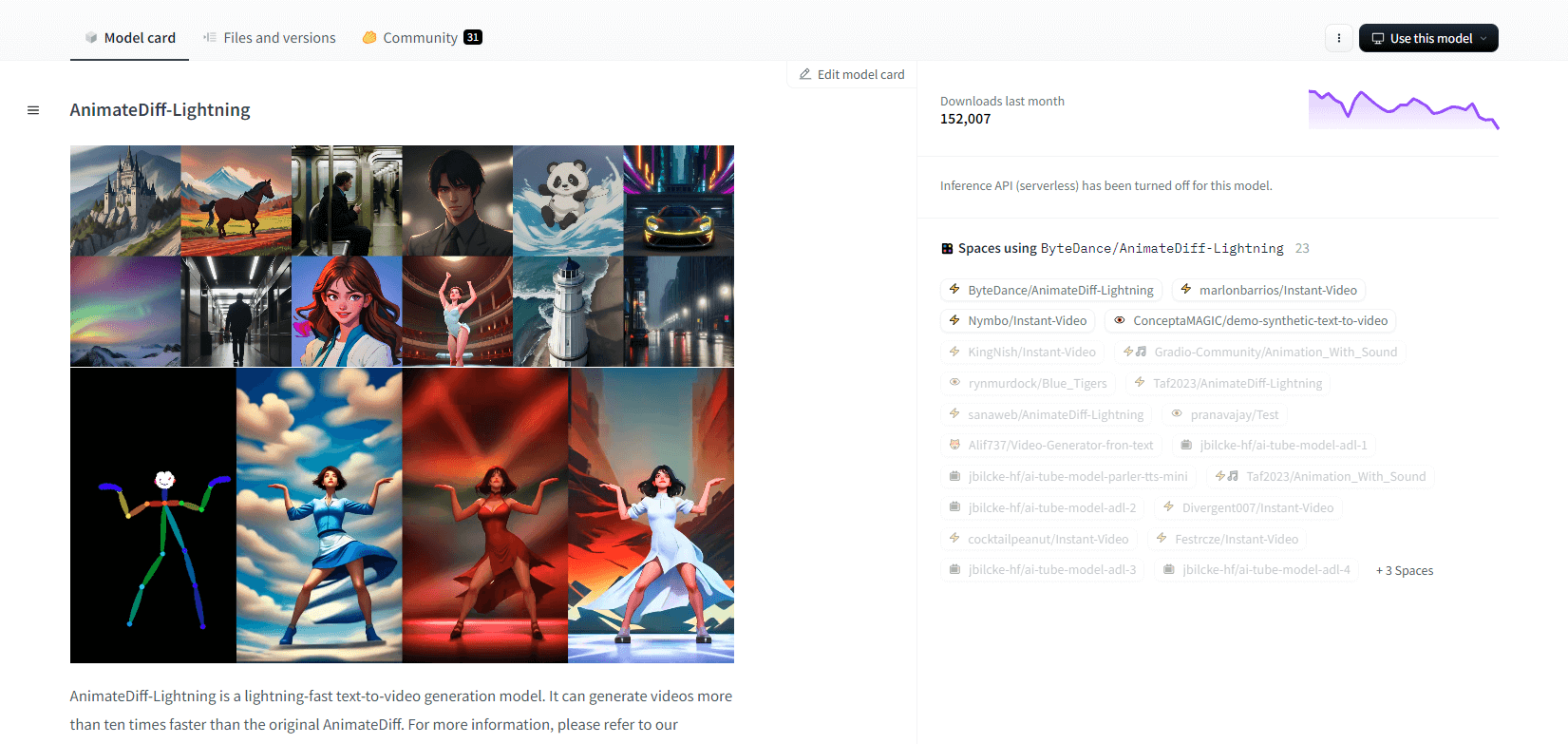
AnimateDiff-Lightning is a cutting-edge, text-to-video generation model that offers speed improvements over the original AnimateDiff, generating videos more than ten times faster. Developed from AnimateDiff SD1.5 v2, this model is available in 1-step, 2-step, 4-step, and 8-step versions, with higher-step models offering superior quality. It excels when used with stylized base models like epiCRealism and Realistic Vision, as well as anime and cartoon models such as ToonYou and Mistoon Anime.
Users can achieve optimal results by experimenting with different settings, such as using Motion LoRAs. For implementation, the model can be used with Diffusers and ComfyUI. It supports video-to-video generation with ControlNet for enhanced output. For more details and a demo, users are encouraged to refer to the research paper: AnimateDiff-Lightning: Cross-Model Diffusion Distillation
Key Features
- Lightning-fast video generation
- Distilled from AnimateDiff
- Multiple-step checkpoints
- High generation quality
- Supports stylized models
- Motion LoRAs support
- Realistic and cartoon options
- Video-to-video generation
Zeroscope V2
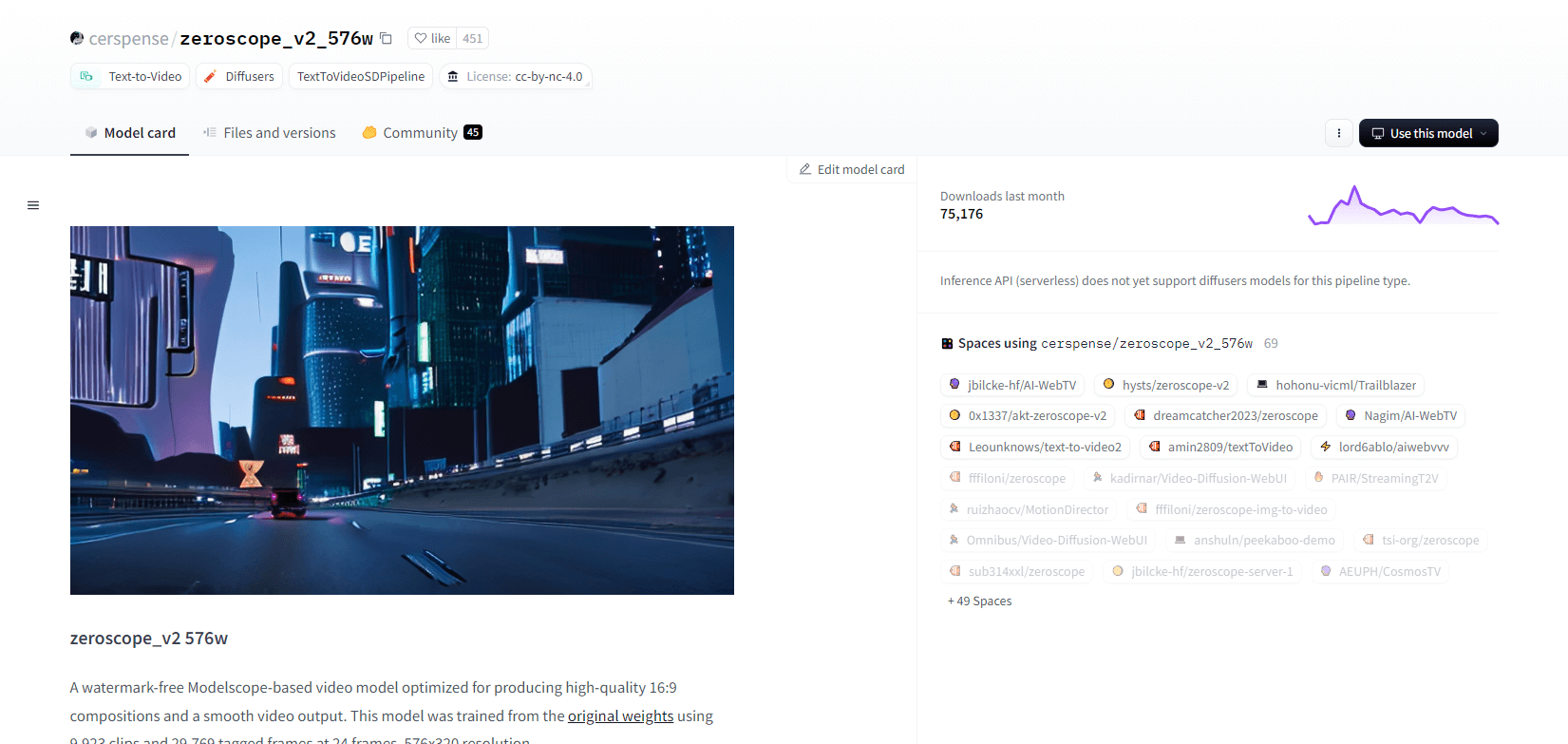
The Modelscope-based zeroscope_v2_567w video model excels at creating high-quality 16:9 videos without watermarks. Trained on 9,923 clips and 29,769 tagged frames at 576x320 resolution and 24 frames per second, it’s ideal for smooth video output. This model is designed for initial rendering before upscaling with zeroscope_v2_XL using vid2vid in the 1111 text2video extension, allowing efficient exploration at lower resolutions.
Upscaling to 1024x576 provides superior compositions. It uses 7.9GB of VRAM for rendering 30 frames. To use, download and replace files in the appropriate directory.
For best results, upscale using zeroscope_v2_XL with a denoise strength between 0.66 and 0.85. Known issues include suboptimal output at lower resolutions or fewer frames. The model can be easily integrated using Diffusers with simple installation and video generation steps.
Key Features
- Watermark-free output
- High-quality 16:9
- Smooth video output
- 576x320 resolution
- 24 frames per second
- Efficient upscaling
- 7.9GB VRAM usage
- Easy integration
VGen
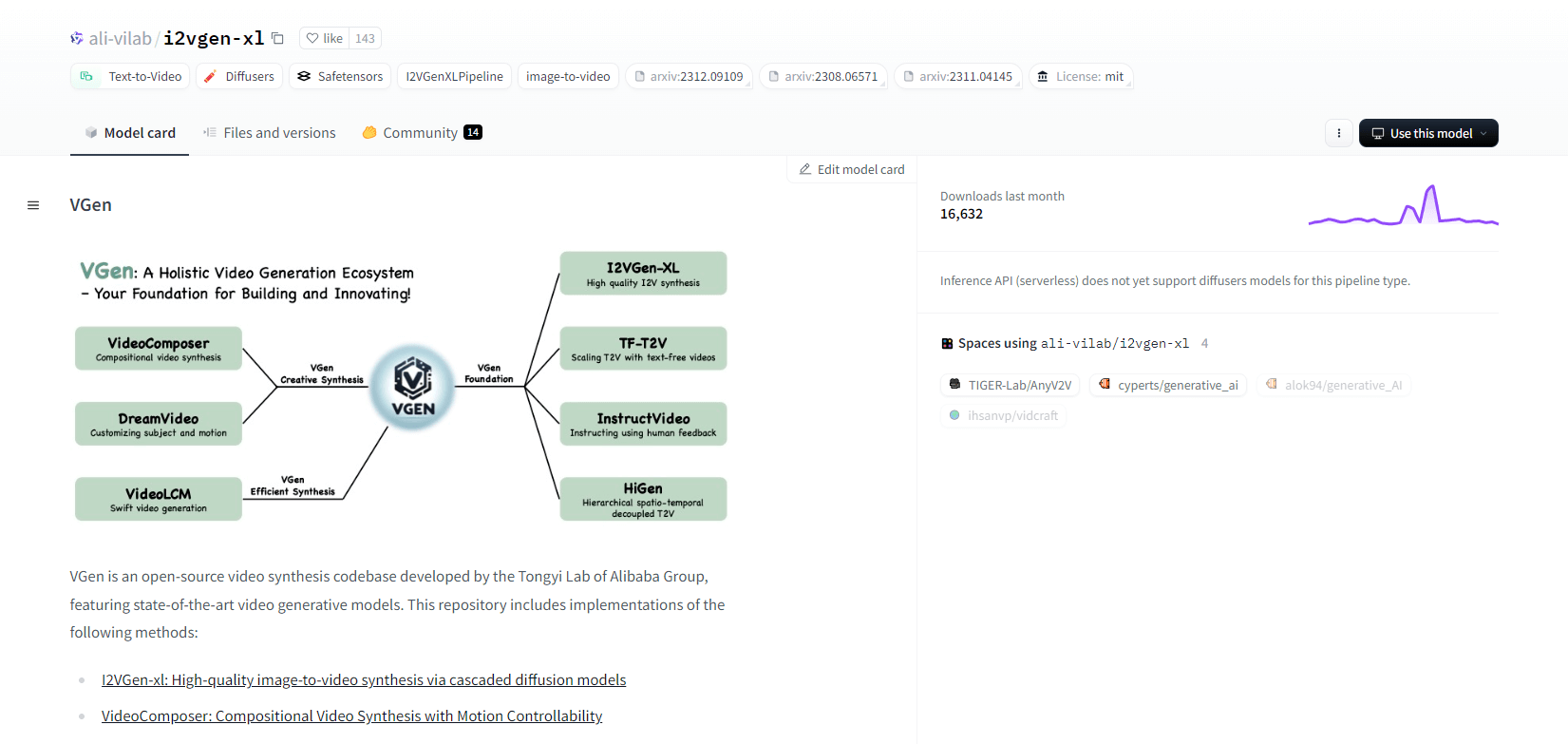
VGen, an open-source video synthesis codebase from Alibaba’s Tongyi Lab, offers advanced video generative models. It includes methods like I2VGen-xl for high-quality image-to-video synthesis, VideoComposer for motion-controllable video synthesis, and more. VGen can create high-quality videos from text, images, desired motions, subjects, and feedback signals. The repository features tools for visualization, sampling, training, inference, and joint training using images and videos. Recent updates include VideoLCM, I2VGen-XL, and the DreamVideo method.
VGen excels in expandability, performance, and completeness. Installation involves setting up a Python environment and necessary libraries. Users can train text-to-video models and run I2VGen-XL for high-definition video generation. Demo datasets and pre-trained models are available to facilitate experimentation and optimization. The codebase ensures easy management and high efficiency in video synthesis tasks.
Key Features
- Open-source codebase
- High-quality synthesis
- Motion controllability
- Text-to-video generation
- Image-to-video conversion
- Expandable framework
- Pre-trained models
- Comprehensive tools
Hotshot XL
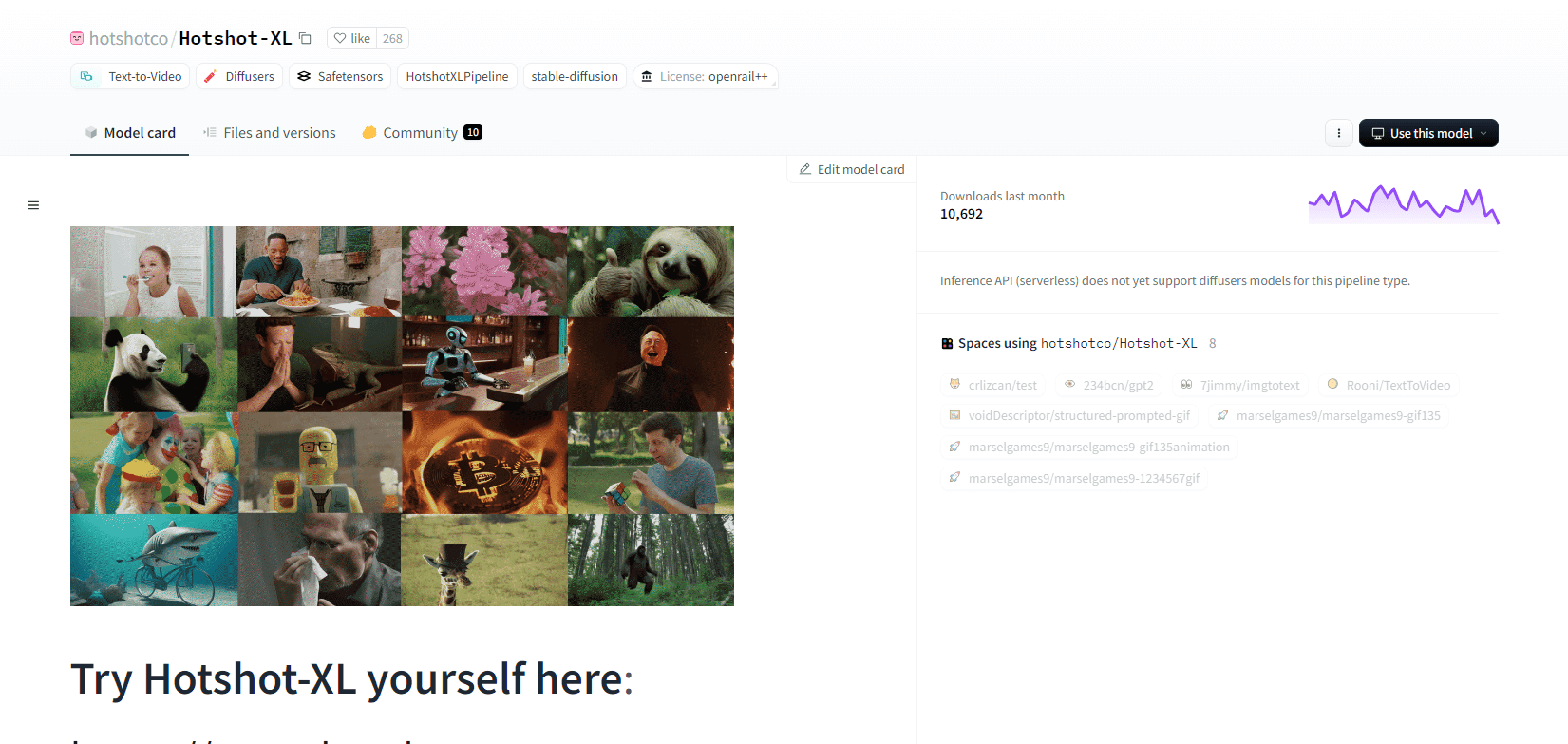
Hotshot-XL, an AI text-to-GIF model developed by Natural Synthetics Inc., operates seamlessly with Stable Diffusion XL. It enables GIF generation using any fine-tuned SDXL model, simplifying the creation of personalized GIFs without additional fine-tuning. This model excels in generating 1-second GIFs at 8 frames per second across various aspect ratios. It leverages Latent Diffusion with pre trained text encoders (OpenCLIP-ViT/G and CLIP-ViT/L) for enhanced performance.
Users can modify GIF compositions using SDXL ControlNet for customized layouts. While capable of versatile GIF creation, Hotshot-XL faces challenges with photorealism and complex tasks like rendering specific compositions. The model's implementation aims to integrate smoothly into existing workflows and is available for exploration on GitHub under a CreativeML Open RAIL++-M License.
Key Features
- Text-to-GIF generation
- Works with SDXL
- Generates 1-second GIFs
- Supports various aspect ratios
- Uses Latent Diffusion
- Pretrained text encoders
- Customizable with ControlNet
- Available on GitHub
Future Capabilities Of Text To Video AI Models
Platforms like Hugging Face are leading the way in developing advanced models that can transform text into high-quality, dynamic video content. This leads us to an assumption that - the future capabilities of text-to-video AI models are truly exciting.
These models are poised to revolutionize content creation, making it faster, more efficient, and more accessible than ever before. By simply inputting a text prompt, users will be able to generate engaging, customized videos that bring their ideas to life.
The potential applications are vast - from marketing and advertising to education and entertainment. Imagine being able to create professional-grade explainer videos or animated stories at the click of a button. The time and cost savings for businesses and creators will be substantial.
Moreover, as these text-to-video models continue to improve in terms of realism, coherence, and flexibility, the quality of the output will become increasingly indistinguishable from human-created video. This democratization of video production will empower more people to share their stories and ideas with the world.
The future of content creation is undoubtedly text-to-video, and Hugging Face is at the forefront of this transformative technology. Prepare to be amazed as these models push the boundaries of what's possible.
Wrapping Up
In conclusion, Huggingface offers a diverse range of models for text-to-video tasks, each bringing unique strengths in generating dynamic visual content from textual descriptions. Whether you prioritize precision, creativity, or scalability, these models provide robust solutions for various applications, promising advancements in AI-driven video synthesis.